Included AI Models
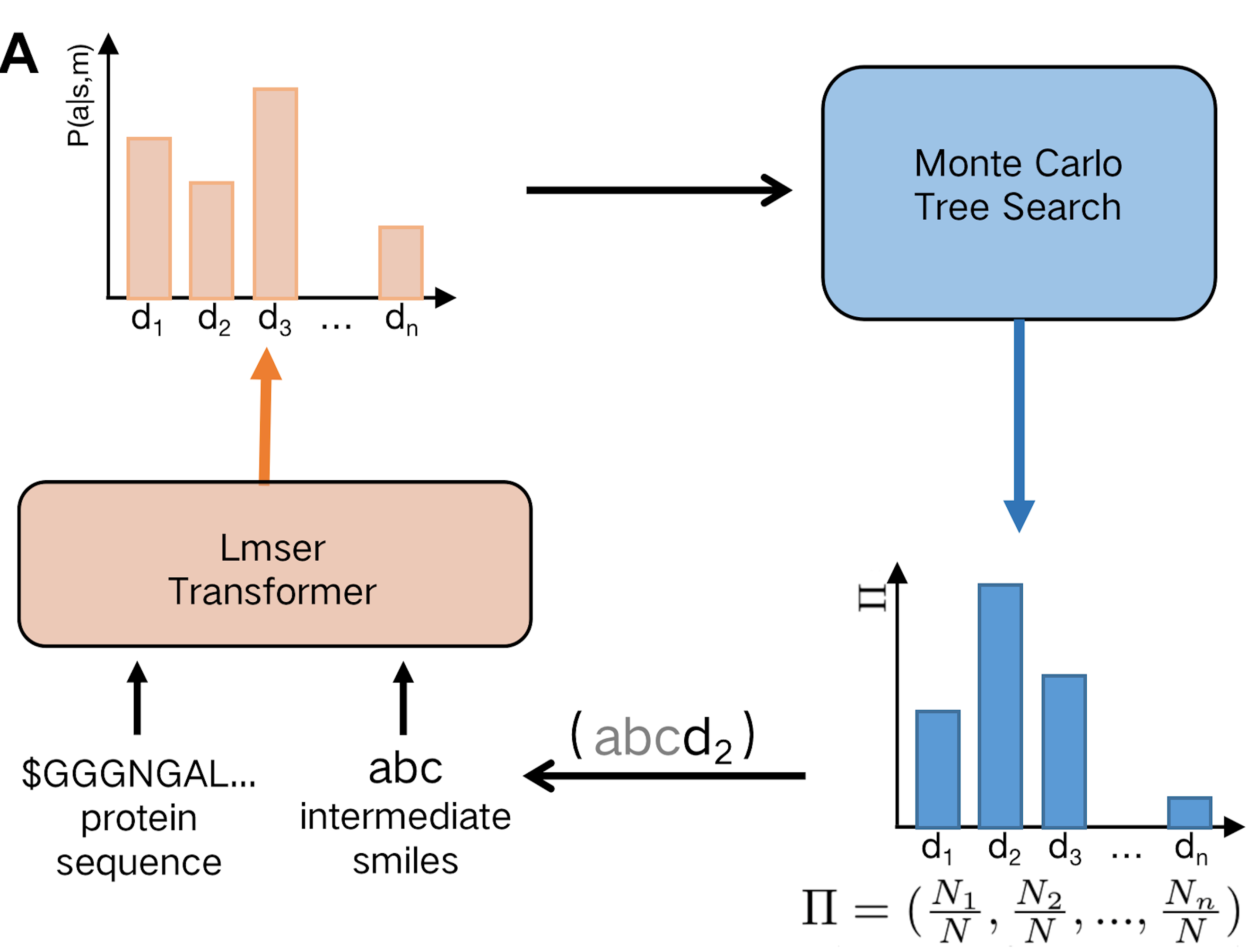
AlphaDrug
AlphaDrug is a de novo drug design approach that uses an improved transformer network and Monte Carlo tree search (MCTS) algorithm to automatically generate molecular drug candidates with high binding affinity for a given protein.
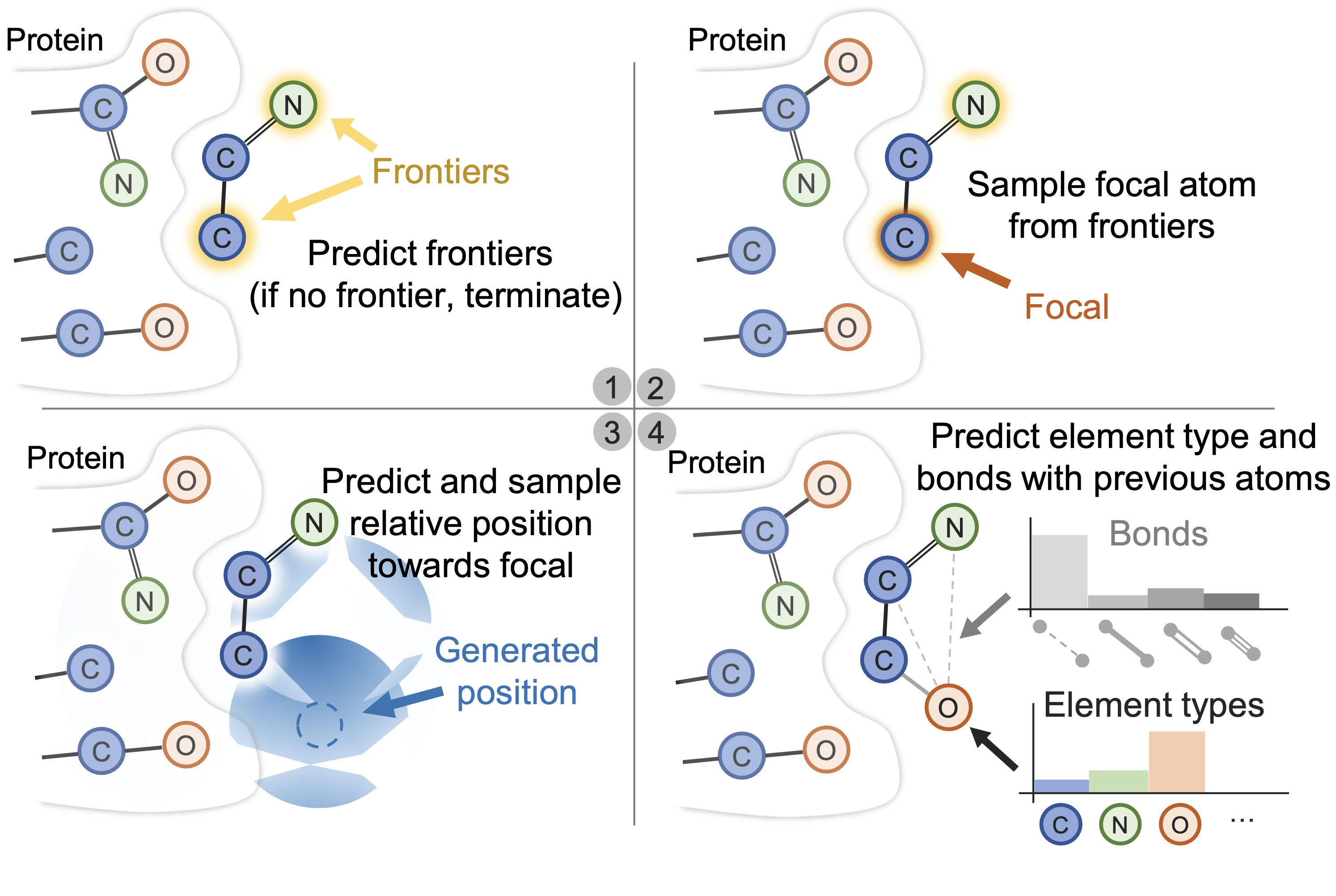
Pocket2Mol
Pocket2Mol consists of two parts: 1) a GNN that captures the spatial and bonding relationships between bound pocket atoms; 2) an 3D coordinate sampling algorithm that samples new 3D drug candidates without relying on MCMC. The molecules produced by Pocket2Mol not only exhibit better affinity and chemical properties but also have more realistic and accurate structures.
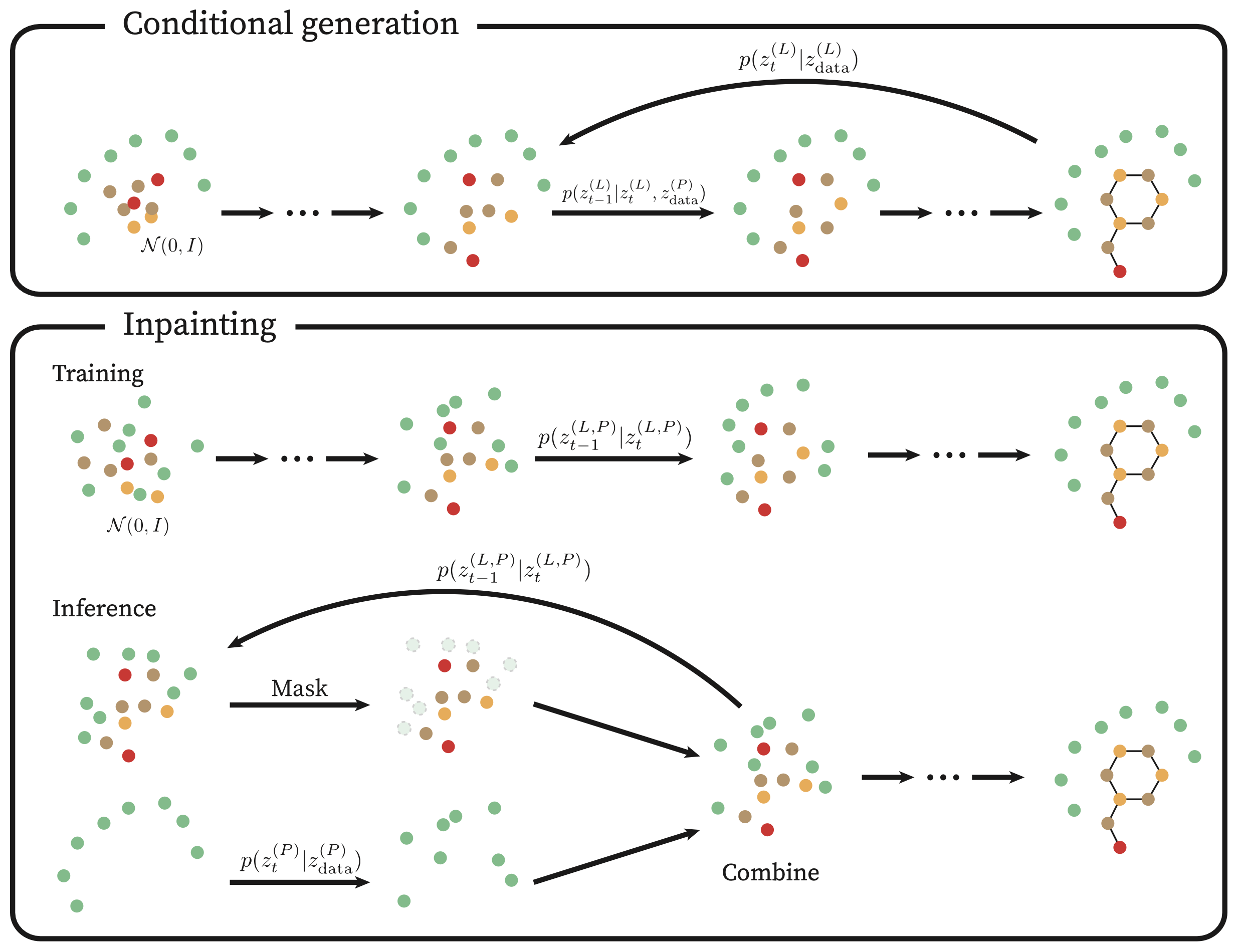
DiffSBDD
DiffSBDD is also a diffusion-based model which utilizes an E(3)-equivariant GNN to learn the denoising process. Two strategies are proposed to generate novel molecules conditioning on protein pockets: protein-conditioned generation and ligand-inpainting generation. The former treats proteins as a fixed environment, while the latter mimics the joint distribution of protein-ligand complexes and inserts new ligands during inference.
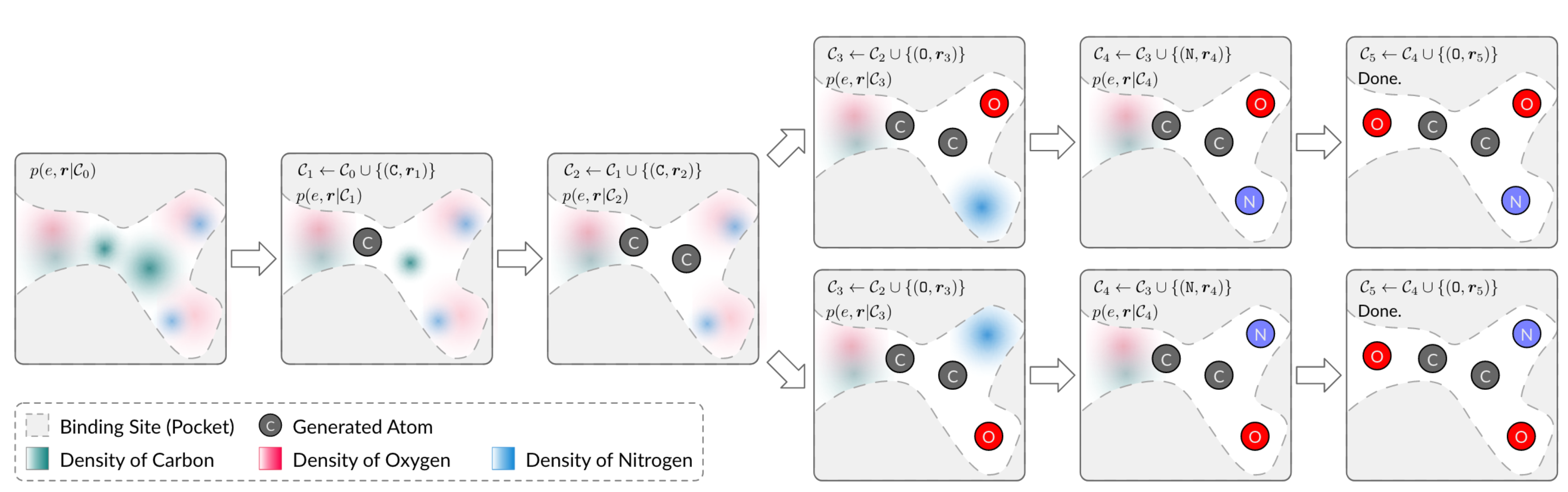
SBDD
SBDD can estimate the probability distribution of atomic positions in 3D space. The auto-regressive sampling algorithm generates 3D molecules by starting with a context that only consists of binding sites. It samples one atom from the distribution at each step and adds it to the context for the next step until there is no space for new atoms.
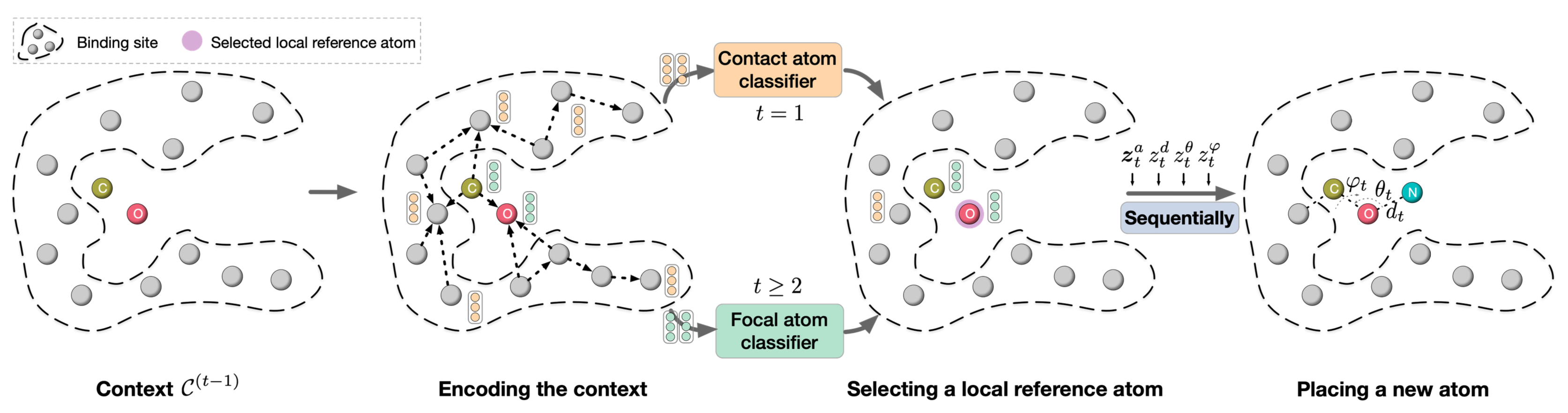
GraphBP
GraphBP is a 3D molecule generation model that uses a GNN to extract intermediate contextual information at each step of the generation process. GraphBP generates atomic type and relative position with respect to the local reference atom to place new atoms, capturing underlying dependencies to generate the protein-ligand complexes' 3D geometry and chemical interactions without discretization of 3D space.
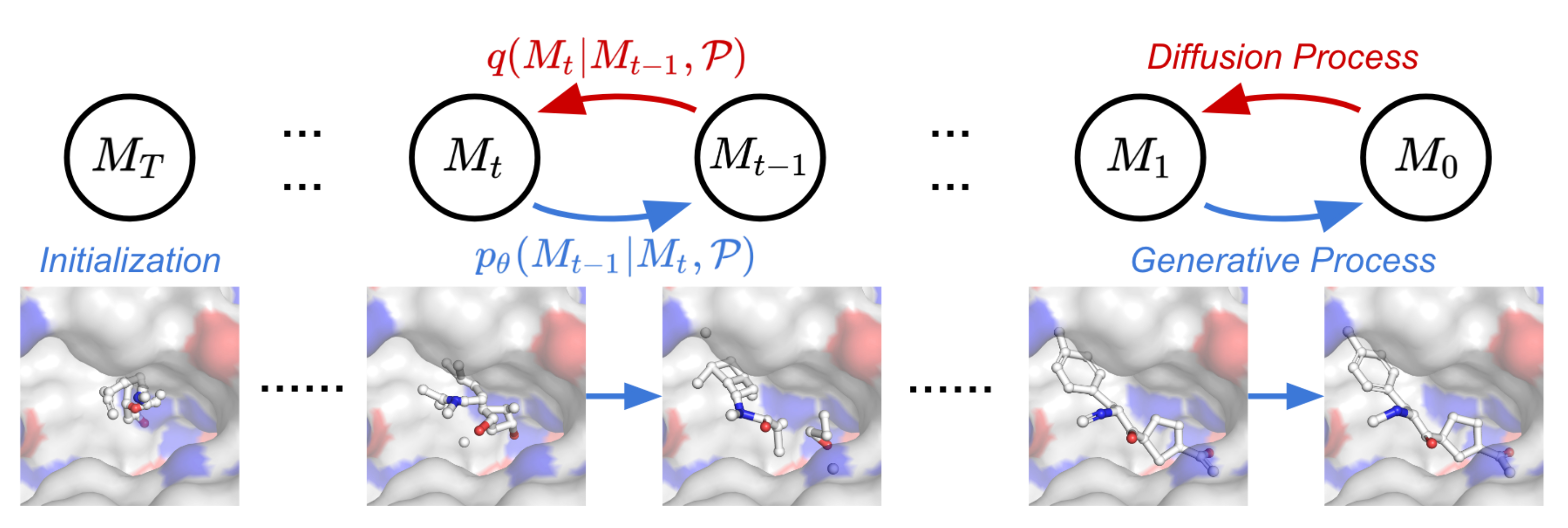
TargetDiff
TargetDiff is a non-autoregressive diffusion model that generates target-sensing molecules. It learns the denoising process of atomic coordinates and types through an E(3) equivariant network, leveraging atomic-scale context. The model is invariant with respect to binding complexes, and can extract representative features from the input molecule with only one forward pass, which can estimate the binding affinity between molecules and the protein of interest.
Call For Models
If your work has not been curated in our database, we invite you to kindly get in touch with us. We will be pleased to consider the inclusion of your model in our database, without any cost.
Call To Action